justified in areas as crucial to the life of an individual as access to credit, employment, accommodation, justice and health. Equity, Bias and Discrimination The obscure nature of this technology is all the more worrying as it may conceal the origins of reported bias, so that we are unable to tell, for example, whether it originates from the algorithm itself or the data used to train it… or both. For instance, some researchers have established the algorithms used by Google in its targeted advertising are more likely to offer less well-paying jobs to women, that YouTube’s moderating algorithms are sometimes slow to react when a harmful content is reported and thus allow its viral spread, or alternatively that algorithms that predict criminal behavior recommend a higher level of surveillance in poorer Afro-American quarters. Indeed, all these algorithms only reproduce the prejudice that already exists in the data they are supplied with. But these observations give rise to legitimate fears, and if we are slow to act we run the risk of seeing a widespread distrust of AI on the part of the general public, which in the long run is liable to curb its development and all the benefits it could bring. The law prohibits any form of discrimination based on exhaustive lists of criteria in the spheres of employment, housing, education and access to goods and services. In these instances, what constitutes discrimination is deemed to be clauses, criteria or practices which seem to be harmless, but which are liable to leave certain individuals at a disadvantage compared to others, except where there is objective justification for these clauses, criteria or practices in the form of a legitimate aim and where the means to achieve this aim are appropriate and necessary. The use of deep learning algorithms, which feed off data for the purposes of personalization and assistance with decision-making, has given rise to the fear that social inequalities are being embedded in decision algorithms. In fact, much of the recent controversy surrounding this issue concerns discrimination towards certain minorities or based on gender (particularly black people, women and people living in Because systems that deprived areas). American experience has also incorporate AI technology brought us several similar examples of the effects of discrimination in the field of crime are invading our daily lives, prevention. we legitimately expect Because systems that incorporate AI them to act in accordance technology are invading our daily lives, we with our laws and social legitimately expect them to act in accordance with our laws and social standards. It is standards therefore essential that legislation and ethics control the performance of AI systems. Since we are currently unable to guarantee a priori the performance of a machine learning system (the formal certification of machine learning is still currently a subject of research), compliance with this requirement necessitates the development of procedures, tools and methods which will allow us to audit these systems in order to evaluate their conformity to our legal and ethical frameworks. This is also vital in case of litigation between different parties who are objecting to decisions taken by AI systems. 116
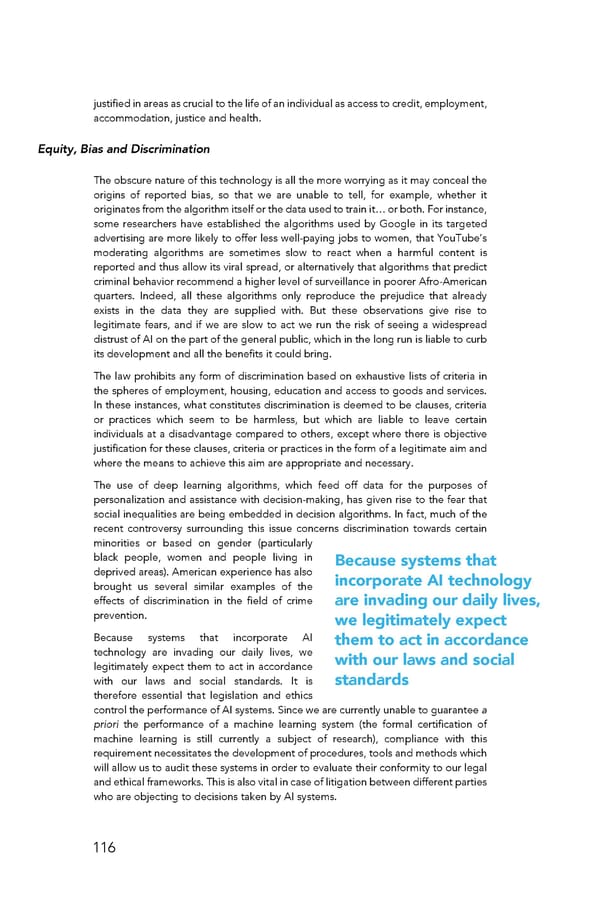