Part 5 — What are the Ethics of AI? so as to arrive at a conclusion (for example, if your income is less than so much per month, you will be refused a loan). Explaining the Decisions Made by Machine Learning Systems The most efficient machine learning technique today, deep neural networks (Deep Learning), does not rely on rules established in advance. In the case of image recognition, for example: if we wanted to develop an algorithm which automatically categorized photos of cats and dogs, the data being processed would consist of images in the form of an array of pixels and it is virtually The accountability of impossible to write out a programme by hand that is systems based on sufficiently powerful to classify all the images accurately machine learning from the data, pixel by pixel. constitutes a real At this stage, the accountability of systems based on scientific challenge machine learning thus constitutes a real scientific challenge, which is creating tension between our need for explanations and our interests in efficiency. But although certain models of machine learning are more easily explainable than others (systems based on rules, simple decision trees and Bayesian networks), nowadays their performance does not generally match up to that of deep learning algorithms. What we do not understand about deep learning Neural networks and deep learning techniques are routinely condemned by their users for seeming just like black boxes. This argument can be equally applied to a large number of other machine learning techniques, whether we are talking about Support Vector Machines or random forests (the operational versions of decision trees). The reason is not so much inherent in the nature of the model used but resides more in a failure to produce an intelligible description of the results produced in each case and, in particular, to highlight the most important features of the case in question that have led to these results. This failure is largely due to the dimensions of the spaces in which the data are evolving, which is particularly crucial in the case of deep learning. For example, for image recognition, a deep network inputs images described by thousands of pixels (4K) and typically memorizes hundreds of thousands, even millions, of parameters (network weights), which it then uses to classify unknown images. It is therefore almost impossible to follow the path of the classification algorithm, which involves these millions of parameters, to its final decision. Although in terms of one image, this accountability seems of relatively low importance, it is a lot more crucial in the granting of a loan, for example. In the long term, the accountability of this technology is one of the conditions of its social acceptability. Regarding certain issues, it is even a question of principle: as a society, we cannot allow certain important decisions to be taken without explanation. In fact, without being able to explain decisions taken by autonomous systems, it is difficult to justify them: it would seem inconceivable to accept what cannot be 115
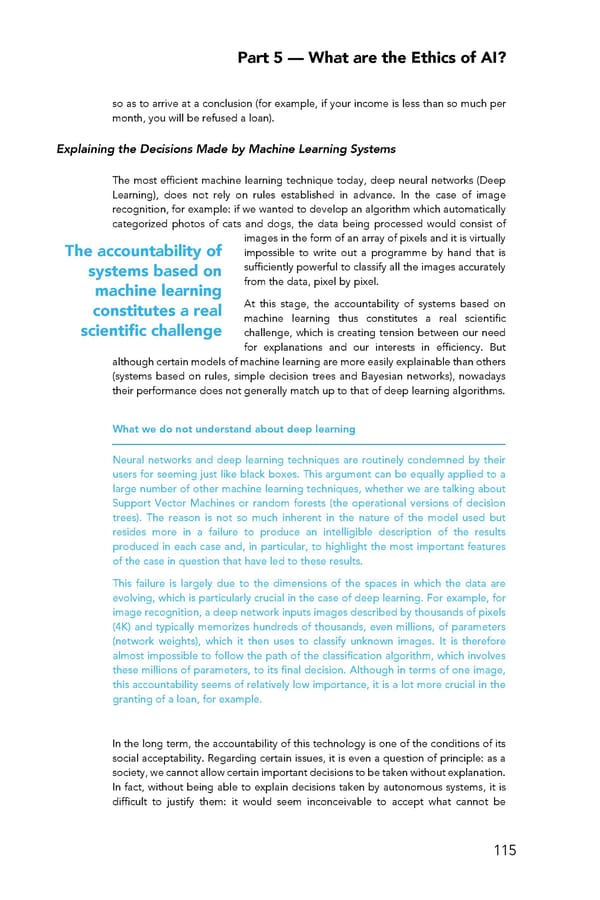