Part 5 — What are the Ethics of AI? To date, these skills—even after the event—are almost non-existent for various reasons. In the first place, deep learning techniques are still too obscure (see above) and their audit protocols are still in their infancy. Then, businesses that have invested substantial sums of money in the construction of their algorithmic systems and would like to reap their rewards are necessarily reluctant to see their intellectual property divulged to third parties. The possibility of accountability for automated decisions is in this sense limited by a certain number of legal obstacles, such as the protection of intellectual property and trade secrets, the protection of personal data, the secrecy necessarily surrounding a certain number of State activities and activities concerned with security and public order. As a result, there is a widespread need to introduce a buffer between the realms of secrecy and of legitimate information. Developing the Auditing of AI Providing official auditing for algorithms The appointment of a body of experts with the requisite skills would appear to be essential to the documentary auditing of algorithms and databases and for checking them using any means deemed necessary. This recommendation is in line with recent developments in the field of competition law and data protection, where the action pursued by the authorities is gradually moving from an a priori control of companies to a logic of audit a posteriori. Such obligations will, where necessary, be laid down by sector-specific regulatory bodies or for specific domains. This recommendation is a response to the specific need for certified audits with probative force when it comes to contentious legal proceedings. To confirm one party’s suspicions or claims, external observations of the performance and effects of algorithms alone are not sufficient to constitute admissible facts in a great number of cases. Whether this occurs during a judicial inquiry or one being carried out by an IAA (an independent administrative authority), it may be necessary to carry out documentary checks. It is not always necessary, useful or even possible to draw conclusions from an examination of a source code. The auditors may be satisfied with simply checking the fairness and equity of a programme (doing only what is required of them), by submitting a variety of false input data, for example, or by creating a large quantity of system user profiles according to precise guidelines, etc. For example, in order to check the gender equity of a recruitment website, a very large number of CVs belonging to men and women who are following the same career paths need to be submitted; in addition, these need to be representative of all those seeking work who are targeted by the site. The output reveals which applications for interview were granted and the average salaries proposed, etc. The system’s provider could be forced to open an API which is designed to test their programme on huge numbers of artificial users (which would also possibly be generated by AI programmes). As regards court referrals, two distinct levels of requirement have been identified: a primary function that could be called upon for legal purposes within the context of investigations carried out by independent administrative authorities, and a secondary function that would follow a referral by the Defender of Rights. 117
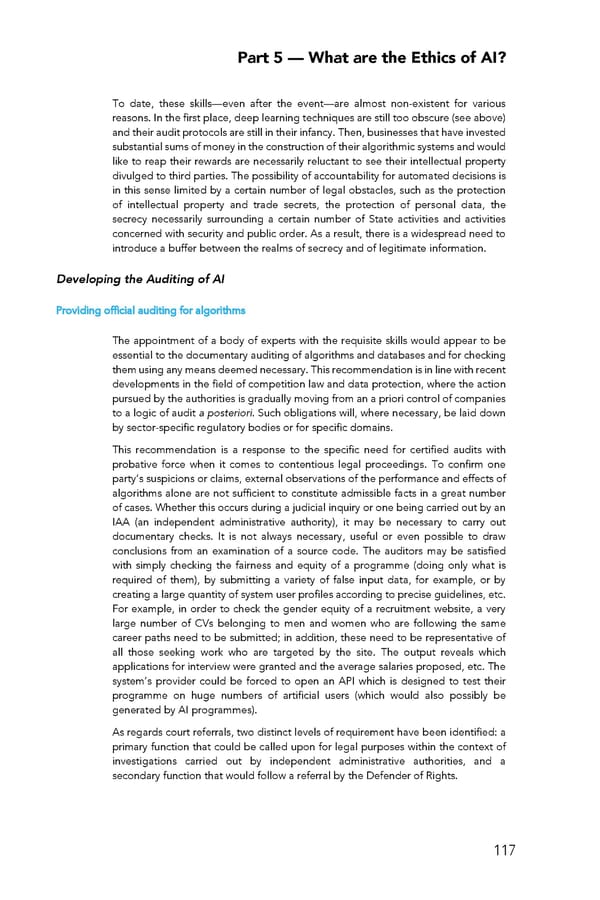