trained to distinguish cats versus dogs, by a physician are transcribed into code if provided a human face picture it will to automatically produce diagnoses. still answer either dog or cat without As an a priori approach, these flinching. techniques require to split into fixed To the other end sits what is called classes the objects processed by the “strong AI”, able to match and even algorithms according to some outperform human capabilities in all predefined features. As such, it is very fields. We are still very far from building challenging to capture the complexity such a machine, and any unconvinced of the real world and establish fixed reader just has to engage in a short categories. discussion with the most advanced Machine learning chatbot to be fully convinced. The fact that the prospect of a “strong AI” Alongside symbolic AI, machine machine is highly unlikely anytime soon learning techniques were developed in does not prevent people, experts and order to model cognitive process regular citizens alike, from passionately directly from previous experience. debating its destructive or positive Every machine learning technique has impact. It is no accident that the sci-fi two steps: the first one is the learning culture, which has been central to the phase that uses the input data (e.g. development of AI, is full of this kind of pictures of cats and dog for the argument. Further, previous classification task) to find the technological advances also raised parameters that best fit the task at similar fears, and it is not surprising that hand. The second step takes the we observe the same phenomenon with learned parameters as input and AI. Nevertheless, policymakers and performs the task accordingly. This is regulators are already urged to the inference phase. consider the fundamental questions brought about by the development of Among machine learning approach, we AI; especially considering diverse can identify a few broad classes of projections regarding its potential problems. dramatic effect on employment. That’s a tricky matter for regulators; little Supervised learning accustomed to such speculative topics. In supervised learning, one learns a set How to build AI? of rules in order to perform a specific task according to a given set of Symbolic AI: grounded on logic examples for which we are given the expected result. For example, we could Symbolic AI refers to a class of be given a million of pictures of cats and approach to AI that aims to reproduce dogs identified as such. The learning the reasoning mechanisms of humans. phase progressively changes the These mechanisms, put in logical form, parameters of the model in order to are produced by a priori modeling of make the error as small as possible on knowledge and rules of logic to perform the known (and unknown) examples. In the task at hand. One of the the inference phase, the algorithm distinguished illustrations of symbolic performs the task on unknown data— AI technique is the expert systems e.g. it classifies dogs and cats on algorithm, capable of producing previously unseen pictures. reasoning from known facts and rules. For instance, diagnostic rules defined
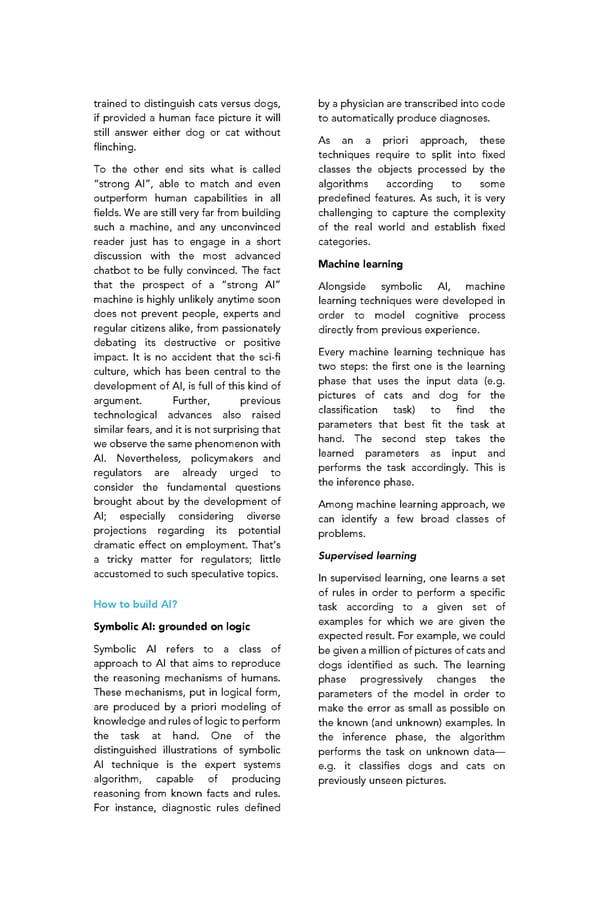