Part 1 — An Economic Policy Based on Data The point of departure for most artificial intelligence strategies thus lies in the accumulation of a large corpus of data. Many of its uses and applications depend directly upon the availability of data; it is, for example, the reason why the automatic processing of the French language is not as advanced as the processing of the English language. It is also the reason why translating from French into English works much better than translating from French into Thai, the corpus of Franco-Thai texts being in shorter supply. The point of departure While raw data is essential, then its value is tenfold when it is structured and annotated6 in such a way for most AI strategies that it can convey information that is recoverable by lies in the accumulation AI techniques. The enhancing and the annotation of of a large corpus of datasets are particularly important for machine learning, but this represents a difficult, time- data consuming and very costly process in terms of both human and financial resources. This is why, in many fields, crowdsourcing (mass outsourcing) is used to collect and especially to annotate this information (particularly through the use of micro- task platforms such as Amazon Mechanical Turk). AI packaged applications generally rely on large bodies of data in the public domain (for example, multilingual texts produced by international organizations are used to improve automatic translation tools); but when it comes to the industrial domain, the onerous tasks of collecting and annotating become a strategic issue. Data constitutes a major competitive advantage in the global competition for AI; from this point of view, it is undeniable that the tech giants have a considerable advantage. However, the volume of data is not everything: smaller datasets (small data) may provide significant results if they are coupled with relevant models. Access to data nevertheless remains an essential condition for the emergence of a French and European AI industry. In an increasingly automated world, not only does public policy and performance of our research depend on this access, but also our collective capacity to determine the way forward for artificial intelligence and the outline of our automated society. However, the current situation in AI is characterized by a critical imbalance between the major stakeholders (the GAFAM7: Google, Amazon, Facebook, Apple and Microsoft, and the BATX: Baidu, Alibaba, Tencent and Xiaomi—whose pre- eminence is entirely due to data collection and recovery) and the rest—businesses and administrations—whose long-term survival is threatened. Associated with this primary imbalance is the secondary, critical one that exists between Europe and the United States. For evidence of this, we only need to look at the flow of data between these huge geographical areas: in France alone, almost 80% of visits to the 25 most popular sites over one month are picked up by the major American platforms8. From this point of view, Europe can be regarded as an exception: both Russia and China, for example, manage to pick up the majority of their users’ data. This is largely due 6. The annotation refers to the addition of information to data describing its content. 7. The acronym varies depending on whether Microsoft and Intel are included, but it still describes a very small number of companies. 8. A study by Cyberstratégie’s Castex Chair: http://www.cyberstrategie.org/?q=fr/flux-donnees 21
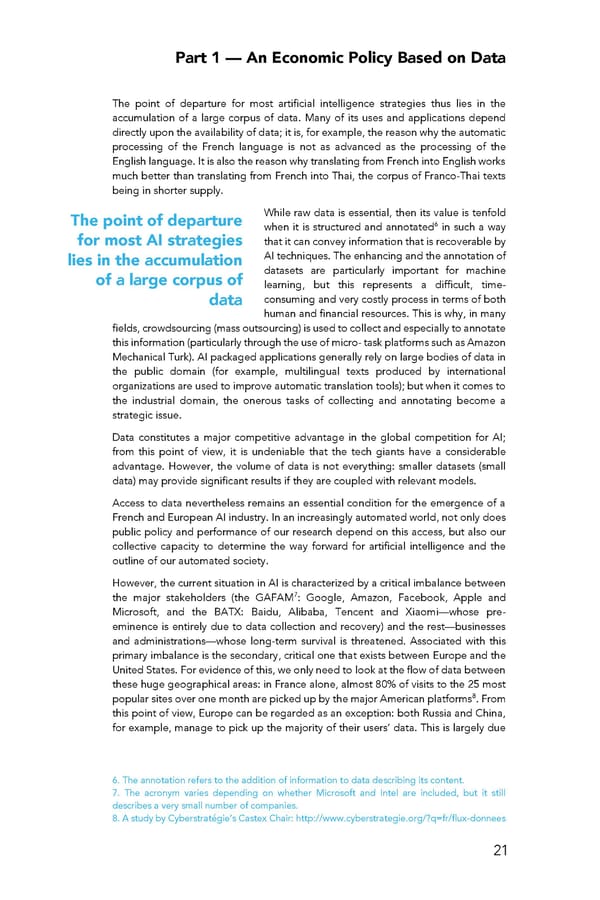