standards of protection. In recent years, the European Union has been committed to consolidating the European market (Digital Single Market) and that is also the purpose of the following propositions. Secondly, efforts made through industrial policy need to be focused on four key areas in the development of AI: health, transport/mobility, environment and defense/security. The suggested measures are particularly aimed at structuring support for innovation around the major challenges of our time, uniting the various ecosystems around sector-specific pooling platforms and making space for experimentation. Here, the role of the State consists in laying the foundation for innovation and providing stakeholders with the means and the resources for breaking new ground, without actually steering the movement in any way. Finally, this is about initiating profound changes in the State, which needs to be a driving force in these transformations. The authorities need to provide themselves with the financial and human resources that will be required in order to incorporate AI into the delivery of its public policies, as much with a view to modernization as to setting an example. This implies making progress in a number of areas, from public procurement to State policy relating to human resources and skills; but it also concerns its approach to innovation itself. This section is the longest, not because it is more important than the others—all these priorities deserve the same amount of attention!—but because the recommendations it contains, particularly those which deal with data, are designed to bolster the others. 1. Reinforcing the European Data Ecosystem The techniques of machine learning signal a break with conventional algorithms, especially because they mark the gradual transition from a programming approach to one that involves learning. This is what led the magazine Wired to predict ‘the end of the code’ in June 2016; in the future, we will no longer programme computers, we will train them instead. The functioning of a machine learning algorithm can be compared to the cognitive development of a child who learns by observing the world around him, by analyzing the way in which individuals interact and by reproducing implicit nonverbal rules. Roughly speaking, machine learning follows the same pattern: algorithms are now trained to learn by themselves without actually being programmed. Rather than programming a car so that it can drive by itself, the manufacturers will for example present it with an infinite number of driving scenarios so that it will be able to take action even in the most unlikely situations5. Data clearly forms the basis for this type of learning. Even though machine learning is not the only expression of artificial intelligence (far from it), it is currently the one which is both the most used, the fastest developing and the most subject to global competition. 5. “At our test site in California, people throw themselves down flat in front of the cars and then curl themselves into a ball”: Chris Urmson, director of the division Google Car (https://www.lesechos.fr/14/03/2016/lesechos.fr/021765692246_comment-la-google-car- utilise-le—deep-learning–.htm) 20
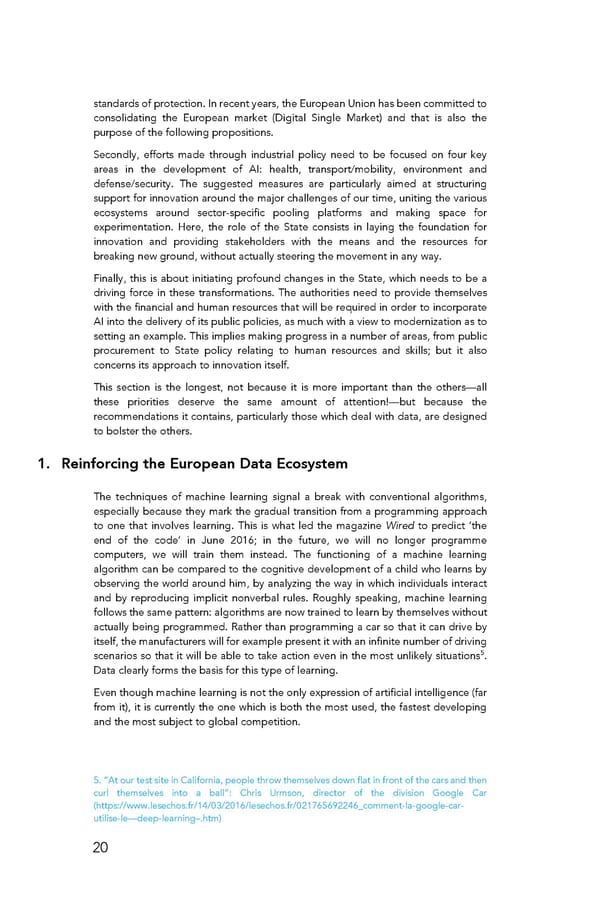