Part 2 — Towards Agile and Enabling Research Some lower-profile areas of AI (such as knowledge representation, the Semantic Web, distributed AI and game theory) should not be overlooked, for they ensure a diversity that must not be forsaken and which perhaps carries the seeds of the next AI revolution. Diversity is all the more important given that the GAFAMs seem to be focusing all their energy on the highest-profile areas, and it is therefore, perhaps, these other areas that we may be able to turn to our advantage, catching these AI giants with all their immense resources by surprise. A series of strategic themes, which will be touched on throughout the report, has to do with ethics and the validation and certification of AI technologies, the aim being confidence on the part of all stakeholders in their results: from validation in terms of theoretical proof to explicability, transparency, causality and fairness. More broadly speaking, theory (and that of deep learning in particular) is lagging behind practice today, and close collaborations with other fields of mathematics and the information & communication sciences and technologies (without really being able to talk about interdisciplinary, then) are to be set up, from game theory to logic and formal proof, from information theory to geometric approaches. In particular, France excels to such an extent in terms of proof of program correctness that a partnership between the two AI and proof communities can only result in major advances being made. Without immediate theoretical progress, it is vital, to ensure swift dissemination of learning techniques, to acquire the means of choosing the right algorithm and then of configuring it, entirely automatically on the basis of data. We are talking about similar research to research in program synthesis here … which, incidentally, currently involves deep neural network approaches as well as other less conventional approaches. Along with image and video analysis and vision processing, natural language processing has most likely gained the most from the arrival of deep learning (machine translation, textual entailment and understanding, generation), and language interfaces are being mooted as interfaces of the future, even though there is still some way to go here, too, before an AI program can pass the Turing test (the 2 examples of the racist rants tweeted by the chatbot Tay again throw into sharp relief the importance and difficulty of research on certification of AI techniques). In more general terms, the whole of the human-machine interface spectrum is already benefiting from the recent leaps forward made in AI, which are paving the way to new fields (other than security) such as Lifelong Learning in an open and uncertain world. And address concerns in the robotics sector, for which very close interaction between researchers from both fields seems necessary. Another crucial area is that of optimization, whether or not in connection with learning. The field of operational research and combinatorial optimization has thus been highlighted by IVADO (Montreal) as one of their three areas of expertise, and one which they therefore distinguish from AI. In any case, the economic repercussions (all of the logistics for starters), and the impacts on AI in general (planning, constraint solving, etc.) are countless. All of the fields making use of 2. "A peine lancée, une intelligence artificielle de Microsoft dérape sur Twitter", LeMonde.fr 24 March 2016, http://lemonde.fr/pixels/article/2016/03/24/a-peine-lancee-une-intelligence- artificielle-de-microsoft-derape-sur-twitter_4889661_4408996.html 65
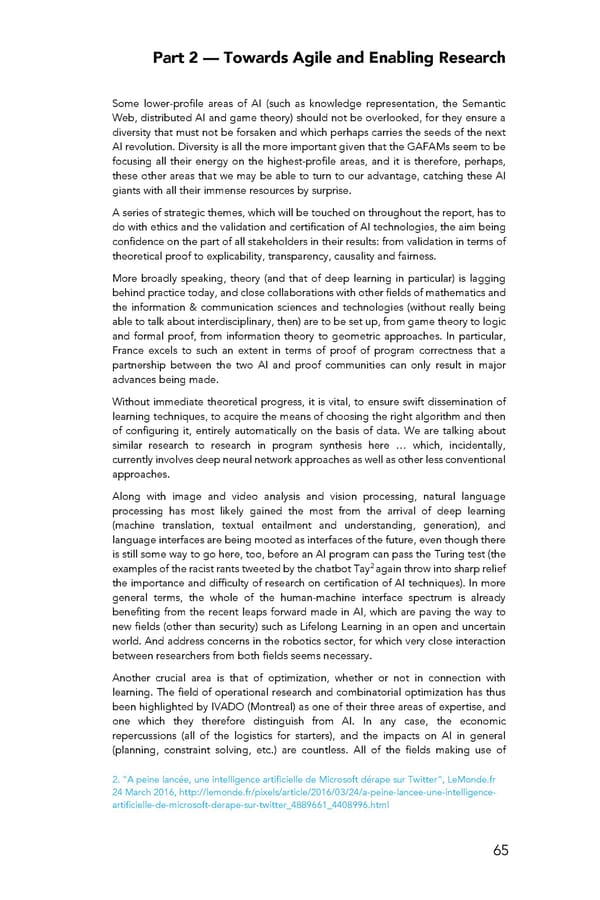