There is still some cause for hope, though: the past few years have marked the end of Moore’s Law, which up until recently guided the components industry’s R&D—a fact that requires the entire ecosystem to reinvent itself and innovate off the beaten track. This opportunity might be made the utmost of in the context of AI in order to produce new approaches, not in a technology race in the sense the term is usually understood, but rather to produce new, innovative, energy-efficient architectures. Among the avenues envisaged, in-memory computing and neuromorphic approaches (see inset) would seem of particular interest. How well a system performs, of course, partly depends on the quality of its components, but not nearly so much as on the system’s architecture as a whole (processors, memory and dataflow in the machine). What is neuromorphic technology? This technology draws its inspiration from the brain’s internal organization and is capable of impressive cognitive tasks with less consumption than a light bulb. We speak of “neuromorphic chips”. Neuromorphic systems are extremely energy- efficient in comparison with processors and graphic cards, due to their exploitation of two strategies. First of all, they bring computing and memory as close together as possible, so limiting data exchanges, which are currently the main source of energy consumption in processors. Secondly, they carry out computing less accurately than processors but in a much more energy-efficient way, either by using low-precision digital circuits (with small numbers of bits) or using the intrinsic nonlinearities in electronic components, which are an essential part of modern approaches such as neural networks. It should be borne in mind, however, that neuromorphic technologies do not necessarily solve all learning problematics. Several articles have tried to quantify energy gains obtained via such technologies: IBM’s TrueNorth neuromorphic chip, for example, consumes 20 mW/cm2 compared with 100 W/cm2 for conventional computers, for implementation of neural networks. Learning is carried out offline, however. Online learning via these technologies remains a challenge our researchers have yet to resolve, and could therefore be the subject of an innovation challenge. Source: The Centre for Nanoscience and Technology’s contribution to the mission. As well as provision of general support to the semiconductor industry, it might well be necessary to organize another innovation challenge bearing on construction of a supercomputer, for example, or embedded means of computing adapted to AI and only requiring European technologies. The aim of such a challenge would be to come up with new architectures taking advantage of European technological innovations—in the fields of in-memory computing or neuromorphics, for example. Such a challenge could well further the development of the transport sector at European level, especially in the event of setup of a European Agency for Disruptive Innovation. 52
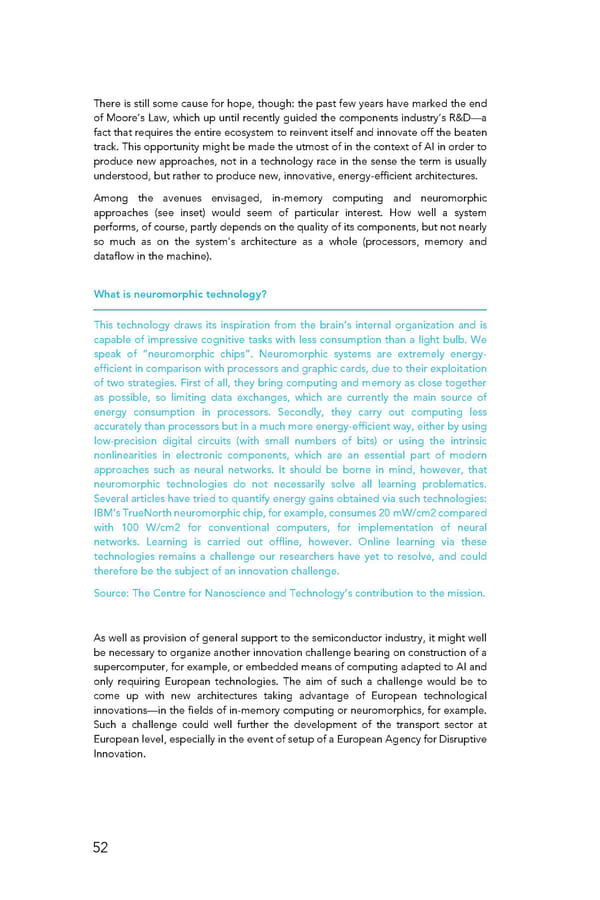